We analyzed temporal trends and significant changes in food consumption clear or availabilityin Bangladesh from 1961 to 2013. Because of the lack dataset national long-term food intake, this study uses data derived from FAO food balance sheet. Joinpoint We used regression analysis to identify significant changes in temporal trends.
The annual percent change (APC) is calculated for each segment of the trend. obviously intake root flour, eggs, fish, vegetables, milk, and vegetable oils are significantly increased (p <0.05) in the diet of Bangladesh since 1961; whereas cereals changed only by 4.65%. Bangladesh has experienced three structural changes in their diet history after the Liberation War, although the level of intake has been woefully inadequate.
Initially, since the late 1970s, it was clear the intake of vegetable oils increased at the market rate (APC = 7:53). Furthermore, since the early 1990s, the real power behind the structural changes in the diet has been a clear trend of increase in the intake of fish (APC = 5:05), eggs (APC = 4.65), and meat (APC = 1.54).
Lastly, since the early 2000s, a clear fruit intake (APC = 20.44), vegetables (APC = 10.58), and milk (APC = 3.55) were significantly increased (p <0.05). And quantifies the results showed a significant secular change in the dietary history of Bangladesh from 1961 to 2013. Bangladesh has undergone structural changes are inadequate but it is significant in the diet in the late 1970s, early 1990s and early 2000s.
Overabundance of cereals and inadequate structural changes in the diet may have led to increased prevalence and emergence overweightness, diet-related non-communicable diseases in Bangladesh.
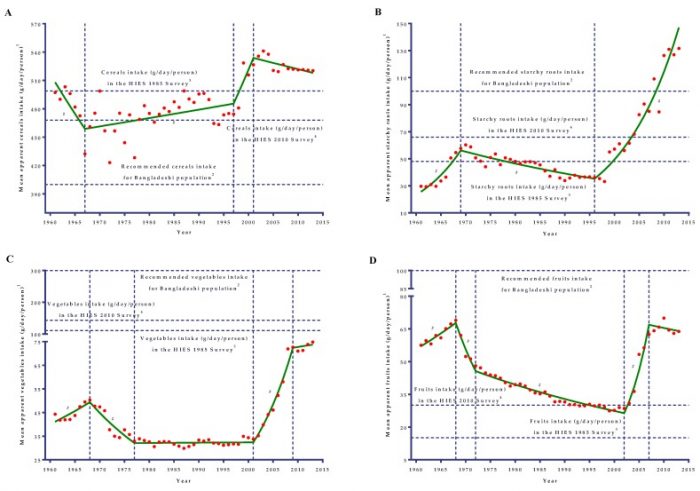
Machine learning in a data-limited regime: Augmenting experiments with synthetic data uncovers order in crumpled sheets.
Machine learning has gained widespread attention as a powerful tool to identify structures within the complex, high-dimensional data. However, this technique as if it does not apply to the experimental system where data is scarce or expensive to obtain. Here, we introduce a strategy to overcome this impasse by adding experimental datasets with synthetic data generated from the system simpler sister.
In particular, we learn spontaneously appear in the local frame crumpled folds of a thin sheet of tissue, the paradigmatic example of spatial complexity, and indicates that the machine learning techniques can be effective even in a regime of limited data.
This is achieved by adding a rare experimental datasets with an inexhaustible amount of simulation data stiff flat sheet is folded, which is simple to simulate and statistical nature of the common stock. This greatly improves the predictive power of the test problem settlement patterns and demonstrated the utility of machine learning in a bench-top experiment where the data is good but it is scarce.